2025
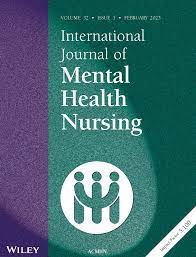
Higgins, Oliver; Wilson, Rhonda L.
In: International Journal of Mental Health Nursing , vol. 34, pp. e70019, 2025.
@article{nokey,
title = {Integrating Artificial Intelligence (AI) With Workforce Solutions for Sustainable Care: A Follow Up to Artificial Intelligence and Machine Learning (ML) Based Decision Support Systems in Mental Health},
author = {Oliver Higgins and Rhonda L. Wilson},
url = {https://onlinelibrary.wiley.com/doi/full/10.1111/inm.70019},
doi = {https://doi.org/10.1111/inm.70019},
year = {2025},
date = {2025-03-08},
urldate = {2025-03-08},
journal = {International Journal of Mental Health Nursing },
volume = {34},
pages = {e70019},
abstract = {This integrative literature review examines the evolving role of artificial intelligence (AI) and machine learning (ML) based clinical decision support systems (CDSS) in mental health (MH) care, expanding on findings from a prior review (Higgins et al. 2023). Using and integrative review framework, a systematic search of six databases was conducted with a focus on primary research published between 2022 and 2024. Five studies met the inclusion criteria and were analysed for key themes, methodologies, and findings. The results reaffirm AI's potential to enhance MH care delivery by improving diagnostic accuracy, alleviating clinician workloads, and addressing missed care. New evidence highlights the importance of clinician trust, system transparency, and ethical concerns, including algorithmic bias and equity, particularly for vulnerable populations. Advancements in AI model complexity, such as multimodal learning systems, demonstrate improved predictive capacity but underscore the ongoing challenge of balancing interpretability with innovation. Workforce challenges, including clinician burnout and staffing shortages, persist as fundamental barriers that AI alone cannot resolve. The review not only confirms the findings from the first review but also adds new layers of complexity and understanding to the discourse on AI-based CDSS in MH care. While AI-driven CDSS holds significant promise for optimising MH care, sustainable improvements require the integration of AI solutions with systemic workforce enhancements. Future research should prioritise large-scale, longitudinal studies to ensure equitable, transparent, and effective implementation of AI in diverse clinical contexts. A balanced approach addressing both technological and workforce challenges remain critical for advancing mental health care delivery.},
keywords = {},
pubstate = {published},
tppubtype = {article}
}
2024
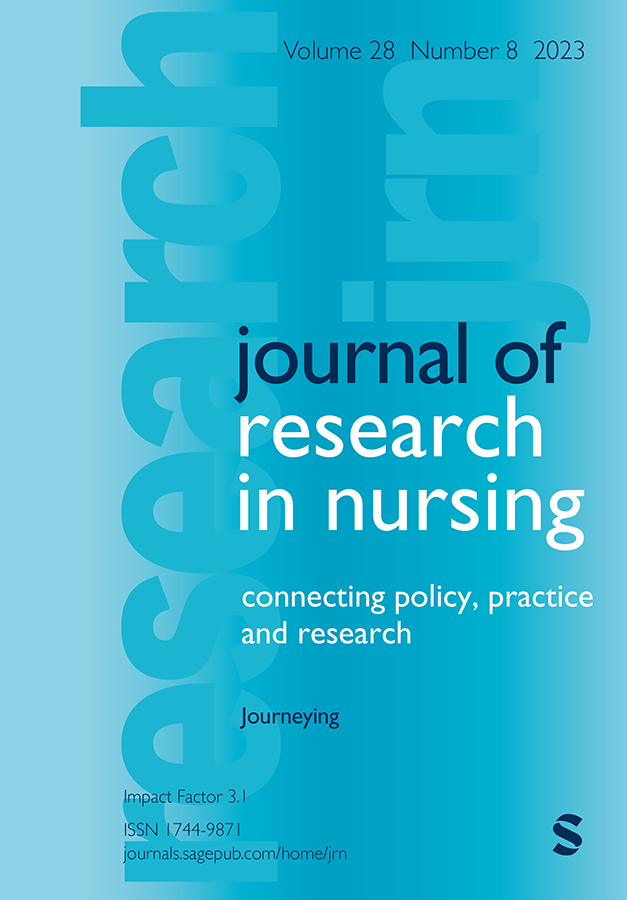
Higgins, Oliver; Chalup, Stephan K; Wilson, Rhonda L
Artificial Intelligence in nursing: trustworthy or reliable? Journal Article
In: Journal of Research in Nursing, vol. 0, iss. 0, 2024.
@article{nokey,
title = {Artificial Intelligence in nursing: trustworthy or reliable?},
author = {Oliver Higgins and Stephan K Chalup and Rhonda L Wilson},
doi = {10.1177/17449871231215696},
year = {2024},
date = {2024-02-29},
urldate = {2024-02-29},
journal = {Journal of Research in Nursing},
volume = {0},
issue = {0},
abstract = {Background:Trustworthiness in Artificial Intelligence (AI) innovation is a priority for governments, researchers and clinicians; however, clinicians have highlighted trust and confidence as barriers to their acceptance of AI within a clinical application. While there is a call to design and develop AI that is considered trustworthy, AI still lacks the emotional capability to facilitate the reciprocal nature of trust.Aim:This paper aims to highlight and discuss the enigma of seeking or expecting trust attributes from a machine and, secondly, reframe the interpretation of trustworthiness for AI through evaluating its reliability and validity as consistent with the use of other clinical instruments.Results:AI interventions should be described in terms of competence, reliability and validity as expected of other clinical tools where quality and safety are a priority. Nurses should be presented with treatment recommendations that describe the validity and confidence of prediction with the final decision for care made by nurses. Future research should be framed to better understand how AI is used to deliver care. Finally, there is a responsibility for developers and researchers to influence the conversation about AI and its power towards improving outcomes.Conclusion:The sole focus on demonstrating trust rather than the business-as-usual requirement for reliability and validity attributes during implementation phases may result in negative experiences for nurses and clinical users.Implications for practice:This research will have significant implications for the way in which future nursing is practised. As AI-based systems become a part of routine practice, nurses will be faced with an increasing number of interventions that require complex trust systems to operate. For any AI researchers and developers, understanding the complexity of trust and creditability in the use of AI in nursing will be crucial for successful implementation. This research will contribute and assist in understanding nurses’ role in this change.},
keywords = {},
pubstate = {published},
tppubtype = {article}
}
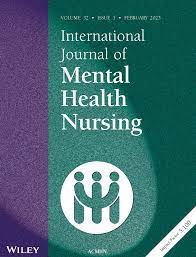
Higgins, Oliver; Sheather‐Reid, Rachel B.; Chalup, Stephan K.; Wilson, Rhonda L.
Disproportionate mental health presentations to emergency departments in a coastal regional community in Australia of first nation people Journal Article
In: International Journal of Mental Health Nursing, 2024, ISSN: 1447-0349.
@article{Higgins2024,
title = {Disproportionate mental health presentations to emergency departments in a coastal regional community in Australia of first nation people},
author = {Oliver Higgins and Rachel B. Sheather‐Reid and Stephan K. Chalup and Rhonda L. Wilson},
doi = {10.1111/inm.13362},
issn = {1447-0349},
year = {2024},
date = {2024-05-26},
urldate = {2024-05-26},
journal = {International Journal of Mental Health Nursing},
publisher = {Wiley},
abstract = {Emergency department (ED) presentations for mental health (MH) help‐seeking have been rising rapidly in recent years. This research aims to identify the service usage demographic for people seeking MH care in the ED, specifically in this case, to understand the usage by First Nation people. This retrospective cohort study examined the sociodemographic and presentation characteristics of individuals seeking MH care in two EDs between 2016 and 2021. Data were collected using existing records and analysed using descriptive univariate analysis with statistical significance between the two sites determined using chi‐squared test, p< 0.05. The overall data presented in this analysis show an overall ED mental health presentation rate of 12.02% for those who identified as ‘Aboriginal but not Torres Strait Islander origin’, 0.36% as ‘Both Aboriginal and Torres Strait Islander’ and 0.27% as ‘Torres Strait Islander’ totalling 12.63%. This is an overrepresentation compared to the regional population of 4.9%. One site recorded 14.1% of ED presentations that identified as Aboriginal and/or Torres Strait Islander, over double the site's demographic of 6.3%. Given the disproportionately high representation of First Nation people in MH‐related ED presentations, further research is required to prioritise a First Nation research perspective that draws on First Nation research methods, such as yarning and storytelling to understand the unique cultural needs and challenges experienced by First Nation people accessing MH care via ED. Understanding the demographic is but one step in supporting the Cultural Safety needs of First Nation people. Additionally, research should be designed, governed and led by First Nation researchers.},
keywords = {},
pubstate = {published},
tppubtype = {article}
}
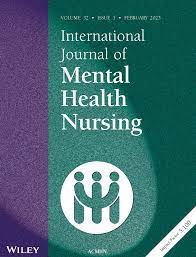
Higgins, Oliver; Chalup, Stephan K.; Wilson, Rhonda L.
Machine Learning Model Reveals Determinators for Admission to Acute Mental Health Wards From Emergency Department Presentations Journal Article
In: International Journal of Mental Health Nursing, pp. 1-16, 2024, ISSN: 1445-8330.
@article{nokey,
title = {Machine Learning Model Reveals Determinators for Admission to Acute Mental Health Wards From Emergency Department Presentations},
author = {Oliver Higgins and Stephan K. Chalup and Rhonda L. Wilson},
url = {https://onlinelibrary.wiley.com/doi/10.1111/inm.13402},
doi = {10.1111/inm.13402},
issn = {1445-8330},
year = {2024},
date = {2024-08-29},
urldate = {2024-08-29},
journal = {International Journal of Mental Health Nursing},
pages = {1-16},
abstract = {This research addresses the critical issue of identifying factors contributing to admissions to acute mental health (MH) wards for individuals presenting to the emergency department (ED) with MH concerns as their primary issue, notably suicidality. This study aims to leverage machine learning (ML) models to assess the likelihood of admission to acute MH wards for this vulnerable population. Data collection for this study used existing ED data from 1 January 2016 to 31 December 2021. Data selection was based on specific criteria related to the presenting problem. Analysis was conducted using Python and the Interpretable Machine Learning (InterpretML) machine learning library. InterpretML calculates overall importance based on the mean absolute score, which was used to measure the impact of each feature on admission. A person's ‘Age’ and ‘Triage category’ are ranked significantly higher than ‘Facility identifier’, ‘Presenting problem’ and ‘Active Client’. The contribution of other presentation features on admission shows a minimal effect. Aligning the models closely with service delivery will help services understand their service users and provide insight into financial and clinical variations. Suicidal ideation negatively correlates to admission yet represents the largest number of presentations. The nurse's role at triage is a critical factor in assessing the needs of the presenting individual. The gap that emerges in this context is significant; MH triage requires a complex understanding of MH and presents a significant challenge in the ED. Further research is required to explore the role that ML can provide in assisting clinicians in assessment.},
keywords = {},
pubstate = {published},
tppubtype = {article}
}
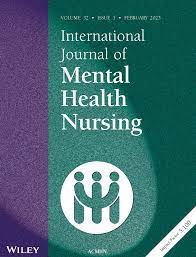
Higgins, Oliver; Sheather‐Reid, Rachel B.; Chalup, Stephan K.; Wilson, Rhonda L.
In: International Journal of Mental Health Nursing, 2024.
@article{nokey,
title = {Sociodemographic Factors and Presentation Features of Individuals Seeking Mental Health Care in Emergency Departments: A Retrospective Cohort Study},
author = {Oliver Higgins and Rachel B. Sheather‐Reid and Stephan K. Chalup and Rhonda L. Wilson},
url = {https://onlinelibrary.wiley.com/doi/10.1111/inm.13414},
doi = {10.1111/inm.13414},
year = {2024},
date = {2024-09-16},
urldate = {2024-09-16},
journal = {International Journal of Mental Health Nursing},
abstract = {Emergency Department (ED) presentations for Mental Health (MH) help-seeking have been rising rapidly, with EDs as the main entry point for most individuals in Australia. The objective of this retrospective cohort study was to analyse the sociodemographic and presentation features of people who sought mental healthcare in two EDs located in a regional coastal setting in New South Wales (NSW), Australia from 2016 to 2021. This article is a part of a broader research study on the utilisation of machine learning in MH. The objective of this study is to identify the factors that lead to the admission of individuals to an MH inpatient facility when they seek MH care in an ED. Data were collected using existing records and analysed using descriptive univariate analysis with statistical significance between the two sites was determined using Chi squared test, p < 0.05. Two main themes characterise dominant help-seeking dynamics for MH conditions in ED, suicidal ideation, and access and egress pathways. The main findings indicate that suicidal ideation was the most common presenting problem (38.19%). People presenting to ED who ‘Did not wait’ or ‘Left at own risk’ accounted for 10.20% of departures from ED. A large number of presentations arrived via the ambulance, accounting for 45.91%. A large proportion of presentations are related to a potentially life-threatening condition (suicidal ideation). The largest proportion of triage code 1 ‘Resuscitation’ was for people with presenting problem of ‘Behavioural Disturbance’. Departure and arrival dynamics need to be better understood in consultation with community and lived experience groups to improve future service alignment with the access and egress pathways for emergency MH care.},
keywords = {},
pubstate = {published},
tppubtype = {article}
}

Higgins, Oliver; Wilson, Rhonda L.; Chalup, Stephan K.
Using machine learning to assist decision making in the assessment of mental health patients presenting to emergency departments Journal Article
In: Digital Health, 2024.
@article{nokey,
title = {Using machine learning to assist decision making in the assessment of mental health patients presenting to emergency departments},
author = {Oliver Higgins and Rhonda L. Wilson and Stephan K. Chalup},
url = {https://journals.sagepub.com/doi/full/10.1177/20552076241287364},
year = {2024},
date = {2024-11-11},
urldate = {2024-11-11},
journal = {Digital Health},
abstract = {Abstract
Objective
The objective of this study was to assess the predictability of admissions to a MH inpatient ward using ML models, based on routine data collected during triage in EDs. This research sought to identify the most effective ML model for this purpose while considering the practical implications of model interpretability for clinical use.
Methods
The study utilised existing data from January 2016 to December 2021. After data pre-processing, an exploratory analysis revealed the non-linear nature of the dataset. Six different ML models were tested: Random Forest, XGBoost, CatBoost, k-Nearest Neighbours (kNN), Explainable Boosting Machine (EBM) using InterpretML, and Support Vector Machine using Support Vector Classification (SVC). The performance of these models was evaluated using various metrics including the Matthews Correlation Coefficient (MCC).
Results
Among the models evaluated, the CatBoost model achieved the highest MCC score of 0.1952, demonstrating superior balanced accuracy and predictive power, particularly in correctly identifying positive cases. The InterpretML model also performed well, with an MCC score of 0.1914. While CatBoost showed strong predictive capabilities, its complexity poses challenges for clinical interpretation. Conversely, the InterpretML model, though slightly less powerful, offers better transparency and is more practical for clinical use.
Conclusion
The findings suggest that the CatBoost model is a compelling choice for scenarios prioritising the detection of positive cases. However, the InterpretML model's ease of interpretation makes it more suitable for clinical application. Integrating explanation methods like SHAP with non-linear models could enhance model transparency and foster clinician trust. Further research is recommended to refine non-linear models within decision support systems, explore multi-source data integration, understand clinician attitudes towards ML, and develop real-time data collection systems. This study highlights the potential of ML in predicting MH admissions from ED data while stressing the importance of interpretability, ethical considerations, and ongoing validation for successful clinical implementation.},
keywords = {},
pubstate = {published},
tppubtype = {article}
}
Objective
The objective of this study was to assess the predictability of admissions to a MH inpatient ward using ML models, based on routine data collected during triage in EDs. This research sought to identify the most effective ML model for this purpose while considering the practical implications of model interpretability for clinical use.
Methods
The study utilised existing data from January 2016 to December 2021. After data pre-processing, an exploratory analysis revealed the non-linear nature of the dataset. Six different ML models were tested: Random Forest, XGBoost, CatBoost, k-Nearest Neighbours (kNN), Explainable Boosting Machine (EBM) using InterpretML, and Support Vector Machine using Support Vector Classification (SVC). The performance of these models was evaluated using various metrics including the Matthews Correlation Coefficient (MCC).
Results
Among the models evaluated, the CatBoost model achieved the highest MCC score of 0.1952, demonstrating superior balanced accuracy and predictive power, particularly in correctly identifying positive cases. The InterpretML model also performed well, with an MCC score of 0.1914. While CatBoost showed strong predictive capabilities, its complexity poses challenges for clinical interpretation. Conversely, the InterpretML model, though slightly less powerful, offers better transparency and is more practical for clinical use.
Conclusion
The findings suggest that the CatBoost model is a compelling choice for scenarios prioritising the detection of positive cases. However, the InterpretML model's ease of interpretation makes it more suitable for clinical application. Integrating explanation methods like SHAP with non-linear models could enhance model transparency and foster clinician trust. Further research is recommended to refine non-linear models within decision support systems, explore multi-source data integration, understand clinician attitudes towards ML, and develop real-time data collection systems. This study highlights the potential of ML in predicting MH admissions from ED data while stressing the importance of interpretability, ethical considerations, and ongoing validation for successful clinical implementation.
2023
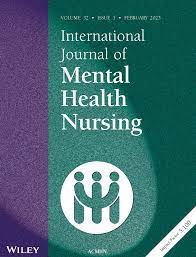
Higgins, Oliver; Short, Brooke L; Chalup, Stephan K; Wilson, Rhonda L
Artificial intelligence (AI) and machine learning (ML) based decision support systems in mental health: An integrative review Journal Article
In: International Journal of Mental Health Nursing, 2023.
@article{nokey,
title = {Artificial intelligence (AI) and machine learning (ML) based decision support systems in mental health: An integrative review},
author = {Oliver Higgins and Brooke L Short and Stephan K Chalup and Rhonda L Wilson},
url = {https://onlinelibrary.wiley.com/doi/10.1111/inm.13114},
doi = {10.1111/inm.13114},
year = {2023},
date = {2023-02-07},
urldate = {2023-02-07},
journal = {International Journal of Mental Health Nursing},
abstract = {An integrative review investigating the incorporation of artificial intelligence (AI) and machine learning (ML) based decision support systems in mental health care settings was undertaken of published literature between 2016 and 2021 across six databases. Four studies met the research question and the inclusion criteria. The primary theme identified was trust and confidence. To date, there is limited research regarding the use of AI-based decision support systems in mental health. Our review found that significant barriers exist regarding its incorporation into practice primarily arising from uncertainty related to clinician's trust and confidence, end-user acceptance and system transparency. More research is needed to understand the role of AI in assisting treatment and identifying missed care. Researchers and developers must focus on establishing trust and confidence with clinical staff before true clinical impact can be determined. Finally, further research is required to understand the attitudes and beliefs surrounding the use of AI and related impacts for the wellbeing of the end-users of care. This review highlights the necessity of involving clinicians in all stages of research, development and implementation of artificial intelligence in care delivery. Earning the trust and confidence of clinicians should be foremost in consideration in implementation of any AI-based decision support system. Clinicians should be motivated to actively embrace the opportunity to contribute to the development and implementation of new health technologies and digital tools that assist all health care professionals to identify missed care, before it occurs as a matter of importance for public safety and ethical implementation. AI-basesd decision support tools in mental health settings show most promise as trust and confidence of clinicians is achieved.},
keywords = {},
pubstate = {published},
tppubtype = {article}
}
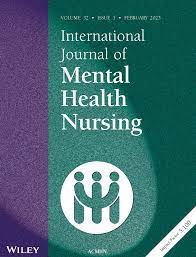
Wilson, Rhonda L; Higgins, Oliver; Atem, Jacob; Donaldson, Andrea E.; Gildberg, Frederik Alkier; Hooper, Mary; Hopwood, Mark; Rosado, Silvia; Solomon, Bernadette; Ward, Katrina; Welsh, Brandi
Artificial intelligence: An eye cast towards the mental health nursing horizon Journal Article
In: International Journal of Mental Health Nursing, 2023.
@article{nokey,
title = {Artificial intelligence: An eye cast towards the mental health nursing horizon},
author = {Rhonda L Wilson and Oliver Higgins and Jacob Atem and Andrea E. Donaldson and Frederik Alkier Gildberg and Mary Hooper and Mark Hopwood and Silvia Rosado and Bernadette Solomon and Katrina Ward and Brandi Welsh},
url = {https://onlinelibrary.wiley.com/doi/10.1111/inm.13121},
doi = {10.1111/inm.13121},
year = {2023},
date = {2023-02-01},
urldate = {2023-02-01},
journal = {International Journal of Mental Health Nursing},
abstract = {Abstract There has been an international surge towards online, digital, and telehealth mental health services, further amplified during COVID-19. Implementation and integration of technological innovations, including artificial intelligence (AI), have increased with the intention to improve clinical, governance, and administrative decision-making. Mental health nurses (MHN) should consider the ramifications of these changes and reflect on their engagement with AI. It is time for mental health nurses to demonstrate leadership in the AI mental health discourse and to meaningfully advocate that safety and inclusion of end users' of mental health service interests are prioritized. To date, very little literature exists about this topic, revealing limited engagement by MHNs overall. The aim of this article is to provide an overview of AI in the mental health context and to stimulate discussion about the rapidity and trustworthiness of AI related to the MHN profession. Despite the pace of progress, and personal life experiences with AI, a lack of MHN leadership about AI exists. MHNs have a professional obligation to advocate for access and equity in health service distribution and provision, and this applies to digital and physical domains. Trustworthiness of AI supports access and equity, and for this reason, it is of concern to MHNs. MHN advocacy and leadership are required to ensure that misogynist, racist, discriminatory biases are not favoured in the development of decisional support systems and training sets that strengthens AI algorithms. The absence of MHNs in designing technological innovation is a risk related to the adequacy of the generation of services that are beneficial for vulnerable people such as tailored, precise, and streamlined mental healthcare provision. AI developers are interested to focus on person-like solutions; however, collaborations with MHNs are required to ensure a person-centred approach for future mental healthcare is not overlooked.},
keywords = {},
pubstate = {published},
tppubtype = {article}
}
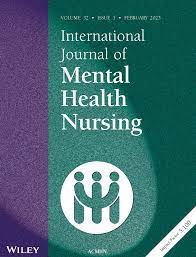
Higgins, Oliver; Wilson, Rhonda L
Commercial determinants and therapeutic chatbots: A mental health nursing perspective Journal Article
In: International Journal of Mental Health Nursing, 2023.
@article{nokey,
title = {Commercial determinants and therapeutic chatbots: A mental health nursing perspective},
author = {Oliver Higgins and Rhonda L Wilson},
url = {https://doi.org/10.1111/inm.13199},
doi = {10.1111/inm.13199},
year = {2023},
date = {2023-08-03},
journal = {International Journal of Mental Health Nursing},
keywords = {},
pubstate = {published},
tppubtype = {article}
}
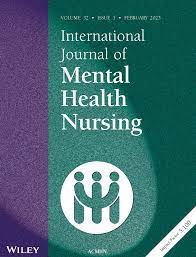
Wilson, Rhonda L; Higgins, Oliver
Editorial: The continued importance of mental health nurses engaging with social media and related emerging technologies Journal Article
In: International Journal of Mental Health Nursing, 2023.
@article{nokey,
title = {Editorial: The continued importance of mental health nurses engaging with social media and related emerging technologies},
author = {Rhonda L Wilson and Oliver Higgins},
url = {https://doi.org/10.1111/inm.13129
},
doi = {10.1111/inm.13129},
year = {2023},
date = {2023-02-14},
urldate = {2023-02-14},
journal = {International Journal of Mental Health Nursing},
keywords = {},
pubstate = {published},
tppubtype = {article}
}
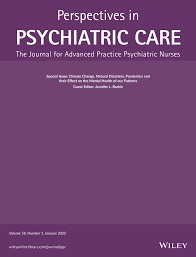
Higgins, Oliver; Short, Brooke L; Chalup, Stephan K; Wilson, Rhonda L
Interpretations of Innovation: The Role of Technology in Explanation Seeking Related to Psychosis Journal Article
In: Perspectives in Psychiatric Care, vol. 2023, 2023.
@article{nokey,
title = {Interpretations of Innovation: The Role of Technology in Explanation Seeking Related to Psychosis},
author = {Oliver Higgins and Brooke L Short and Stephan K Chalup and Rhonda L Wilson},
url = {https://doi.org/10.1155/2023/4464934},
doi = {10.1155/2023/4464934},
year = {2023},
date = {2023-02-08},
urldate = {2023-02-08},
journal = {Perspectives in Psychiatric Care},
volume = {2023},
abstract = {Background. The prominence of technology in modern life cannot be understated. However, for some people, these innovations or their related plausible advancements can be associated with perceptual misinterpretation and/or incorporation into delusional concepts. Objective. This paper aims to explore the intersection of technological advancement and experiencing psychosis. We present a discussion about the explanation seeking that incorporates the concept, that for some people, of technological innovation becoming intertwined with delusional symptoms over the past 100 years. Methods. A longitudinal review of the literature was conducted to synthesize and draw these concepts together, mapping them to a timeline that aligns computing science and healthcare expertise and presents the significant technological changes of the modern era charted against mental health milestones and reports of technology-related delusions. Results. It is possible for technology to be incorporated into the content of delusions with evidence supporting a link between the rate of technological change, the content of delusions, and the use of technology as a way of seeking an explanation. Moreover, analysis suggests a need to better understand how innovations may impact the mental health of people at risk of psychosis and other mental health conditions. Conclusions. Clinical experts and lived experience experts need to be informed about and collaborate with future research and development of technology, specifically artificial intelligence and machine learning, early in the development cycle. This concurs with other artificial intelligence research recommendations calling for design attention to the development and implementation of technological innovation applied in a mental health context.},
keywords = {},
pubstate = {published},
tppubtype = {article}
}
2022
Higgins, Oliver; Chalup, Stephan K; Short, Brooke L; Wilson, Rhonda L
Interpretations of Innovation: The intersection of technological advancement and psychosis (Preprint) Journal Article
In: Journal of Medical Internet Research, 2022.
@article{Higgins_2022,
title = {Interpretations of Innovation: The intersection of technological advancement and psychosis (Preprint)},
author = {Oliver Higgins and Stephan K Chalup and Brooke L Short and Rhonda L Wilson},
url = {https://doi.org/10.2196%2Fpreprints.37033},
doi = {10.2196/preprints.37033},
year = {2022},
date = {2022-02-01},
urldate = {2022-02-01},
journal = {Journal of Medical Internet Research},
publisher = {JMIR Publications Inc.},
abstract = {ABSTRACT
Background:
The prominence of technology in modern life cannot be understated. However, for some people these innovations or their related plausible advancements, can be associated with perceptual misinterpretation and/or incorporation into delusional concepts.
Objective:
This paper aims to explore the intersection of technological advancement and experiencing psychosis. We present a discussion about the explanation seeking that incorporates the concept, that for some people, of technological innovation becoming intertwined with delusional symptoms over the past 100 years.
Methods:
A longitudinal review of the literature was conducted to synthesise and draw these concepts together, mapping them to a timeline that aligns computing science and healthcare expertise and presents the significant technological changes of the modern era charted against mental health milestones and reports of technology-related delusions.
Results:
It is possible for technology to be incorporated in the content of delusions with evidence supporting a link between the rate of technological change, the content of delusions and the use of technology as a way of seeking an explanation. Moreover, analysis suggests a need to better understand how innovations may impact the mental health of people at risk of psychosis and other mental health conditions.
Conclusions:
Clinical experts and lived experience experts need to be informed about and collaborate with future research and development of technology, specifically artificial intelligence and machine learning, early in the development cycle. This concurs with other artificial intelligence research recommendations calling for design attention to the development and implementation of technological innovation applied in a mental health context.},
keywords = {},
pubstate = {published},
tppubtype = {article}
}
Background:
The prominence of technology in modern life cannot be understated. However, for some people these innovations or their related plausible advancements, can be associated with perceptual misinterpretation and/or incorporation into delusional concepts.
Objective:
This paper aims to explore the intersection of technological advancement and experiencing psychosis. We present a discussion about the explanation seeking that incorporates the concept, that for some people, of technological innovation becoming intertwined with delusional symptoms over the past 100 years.
Methods:
A longitudinal review of the literature was conducted to synthesise and draw these concepts together, mapping them to a timeline that aligns computing science and healthcare expertise and presents the significant technological changes of the modern era charted against mental health milestones and reports of technology-related delusions.
Results:
It is possible for technology to be incorporated in the content of delusions with evidence supporting a link between the rate of technological change, the content of delusions and the use of technology as a way of seeking an explanation. Moreover, analysis suggests a need to better understand how innovations may impact the mental health of people at risk of psychosis and other mental health conditions.
Conclusions:
Clinical experts and lived experience experts need to be informed about and collaborate with future research and development of technology, specifically artificial intelligence and machine learning, early in the development cycle. This concurs with other artificial intelligence research recommendations calling for design attention to the development and implementation of technological innovation applied in a mental health context.
2018
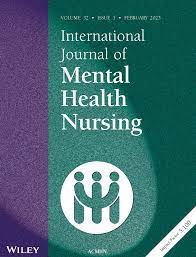
Coates, Dominiek; Woodford, Patricia; Higgins, Oliver; Grover, Deborah
Evaluation of a general practitioner-led cardiometabolic clinic: Physical health profile and treatment outcomes for clients on clozapine Journal Article
In: Int J Ment Health Nurs, vol. 27, no. 1, pp. 303–310, 2018, ISSN: 1447-0349.
@article{pmid28233407,
title = {Evaluation of a general practitioner-led cardiometabolic clinic: Physical health profile and treatment outcomes for clients on clozapine},
author = {Dominiek Coates and Patricia Woodford and Oliver Higgins and Deborah Grover},
doi = {10.1111/inm.12321},
issn = {1447-0349},
year = {2018},
date = {2018-02-01},
urldate = {2018-02-01},
journal = {Int J Ment Health Nurs},
volume = {27},
number = {1},
pages = {303--310},
abstract = {The present study is a review of a cardiometabolic clinic for consumers taking clozapine. This clinic was recently established and co-located with the clozapine clinic at a regional hospital in New South Wales, Australia, to enhance engagement and improve the physical health outcomes of consumers taking antipsychotic medication. A descriptive analysis of clients' (n = 73) information collected during routine care for the first 6 months of the clinic's operation, from January 2016 to July 2016, was conducted. First-visit data were analysed to establish a client profile, consisting of weight, height, blood pressure, pulse, a range of blood measurements, smoking status, alcohol consumption, and eating and exercise habits. Data collected for clients who had three or more visits with the general practitioner (n = 40) were analysed separately for outcomes. Two case studies are used to depict the service received and client profile. At the first appointment, the majority of clients had metabolic syndrome that was mostly left untreated; many of these clients were commenced on metformin. The outcomes are positive, and show that the majority of clients lost weight (82.5%) and had a reduction in body mass index (84.6%); nearly half (44.4%) had a reduction in waist circumference. The majority of clients self-reported increased physical activity (72.5%, n = 29) and positive dietary changes (77.5%, n = 31) since their first appointment. The model trialled by the cardiometabolic clinic integrated a specialist mental health and primary care service, and demonstrates success in engaging clients with severe mental illness in physical health care. Co-location is conceptualized as critical for positive patient outcomes and high levels of engagement.},
keywords = {},
pubstate = {published},
tppubtype = {article}
}
2013
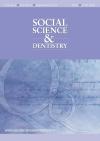
Mullen, Leeann; Higgins, Oliver; Redmayne, Brian; Keegan, Loran; Blinkhorn, Anthony; Blinkhorn, Fiona
The Impact of Dental Disease on a sample of Aboriginal adults living in the Central Coast Region of New South Wales Australia Journal Article
In: Journal of Social Science and Dentistry, vol. 2, iss. 2, pp. 73-79, 2013.
@article{Blinkhorn2013,
title = {The Impact of Dental Disease on a sample of Aboriginal adults living in the Central Coast Region of New South Wales Australia},
author = {Leeann Mullen and Oliver Higgins and Brian Redmayne and Loran Keegan and Anthony Blinkhorn and Fiona Blinkhorn},
url = {https://www.stephenhancocks.com/view.php?article_id=1275&journal_id=147},
year = {2013},
date = {2013-01-01},
urldate = {2013-01-01},
journal = {Journal of Social Science and Dentistry},
volume = {2},
issue = {2},
pages = {73-79},
abstract = {Objective: To record the impact of dental disease on the quality of life of Aboriginal* adults. Setting: An Aboriginal dedicated dental clinic in the Central Coast Region of NSW, Australia. Methods: Adult patients attending the clinic were interviewed about the impact of dental disease on their day to day lives.
Results:
Fifty adults were interviewed of the 61 who attended for care, giving a response rate of 82%. The major impacts were an aching, painful mouth 76%, self conscious about their teeth 62%, embarrassed about their teeth 62%, and diet impacted by poor oral health 64%. Nearly half required the extraction of at least one tooth. Cost was a major barrier for not seeking care for 62% of the participants. Conclusion: Poor oral health impacted on the lives of the Aboriginal respondents, but the offer of free dental care in an Aboriginal dedicated clinic encouraged attendance for treatment, especially amongst women.},
keywords = {},
pubstate = {published},
tppubtype = {article}
}
Results:
Fifty adults were interviewed of the 61 who attended for care, giving a response rate of 82%. The major impacts were an aching, painful mouth 76%, self conscious about their teeth 62%, embarrassed about their teeth 62%, and diet impacted by poor oral health 64%. Nearly half required the extraction of at least one tooth. Cost was a major barrier for not seeking care for 62% of the participants. Conclusion: Poor oral health impacted on the lives of the Aboriginal respondents, but the offer of free dental care in an Aboriginal dedicated clinic encouraged attendance for treatment, especially amongst women.